Opinion
Opinion: Equity, accountability, transparency: Goals for New York City’s new ‘AI czar’
This new role should include focussing on reducing the potential harms of artificial intelligence, misuse and the negative impact of bias in data and algorithms.
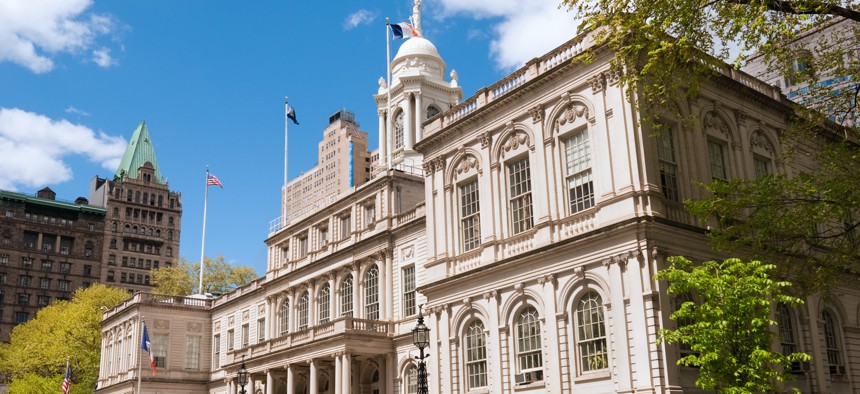
New York City Hall gregobagel-Getty
A Jan. 24 article in City & State discussed New York City’s search for an artificial intelligence “czar” to “supporting agencies’ ‘productive and responsible’ use of artificial intelligence and machine learning tools.”
"Creating governing principles for the responsible use of AI will be part of the new director’s role too, but the city’s technology office isn’t talking about what those standards would be yet,” the article noted. The development of those principles should be the first priority for the new czar. While the city’s AI director role can focus on maximizing the positive impact of artificial intelligence in government agencies, the city must also focus on reducing the potential harms of AI misuse and the negative impact of bias in data and algorithms.
Here at the NYU McSilver Institute’s AI Hub, where I serve as chief AI officer, we are focused on using artificial intelligence and machine learning tools to have a positive impact on underrepresented groups and reducing the harms of these tools on communities of color through both research and policy. A key part of that work is in developing principles and standard best-practices for ethical and equitable AI in a rapidly developing field.
Artificial intelligence and machine learning tools offer the promise of improving everyday New Yorkers’ lives, reducing cost, detecting fraud and predicting where public services can do the most good. But the potential for inequitable and incorrect results remains an ongoing threat.
For example, in a 2019 Berkeley study, a widely used health care prediction tool resulted in a lower standard of care for Black people. In 2016, ProPublica demonstrated that the COMPAS recidivism algorithm’s use in Florida predicted higher risk of recidivism for Black defendants than white defendants, even when controlling for prior crimes, future recidivism, age and gender. Most recently, concerns about bias in hiring and promotion algorithms used in HR decision-making prompted a New York City local law requiring bias audits.
In these examples, the impact of inequitable outcomes from algorithms can cause direct negative impacts, like poor health outcomes, longer sentences and fewer or lost career opportunities. Thus, there is a responsibility to manage the risk of automating bias at scale, particularly at the scale of the New York City government. Defined checks and balances must be a core principle of the city’s AI strategy, and stakeholder input, transparency and clear audit criteria are needed. This is particularly important as artificial intelligence and machine learning tools impact more of our everyday lives.
Equitable Data Methods
If AI is an engine for insight, then the data is the fuel for that engine. If there is bias in the data used to train an algorithm, then it increases the risk that the models will produce biased results. One example is in facial recognition software which may have been trained only on white males but can be inaccurate for females and people of color. The training data should be examined for potential bias and its appropriateness for use across different affected groups.
Transparency & Auditability
With many AI systems, it is unclear how a result was reached. This “black box” effect means even if inputs and outputs are known, what happens inside the system is a mystery. A key part of troubleshooting and correcting biased outcomes is to have a way of tracing and explaining the reasons for an outcome. Audits are important, but also knowing what caused the biased outcome will go a long way to correcting it.
Incorporate Community Engagement
Experts in the field where the algorithms will be applied are critical to reducing algorithmic harm or misuse in everyday life. Too often the models trained for one purpose get reused inappropriately and without input or expertise from the impacted communities. Systems should be designed and deployed with active stakeholder engagement to reduce the risks and maximize the benefits of artificial intelligence and machine learning tools.
These guidelines, while important, are just words until they are put into action. The AI czar must be empowered to have oversight over city artificial intelligence and machine learning tools and the power to enforce standards. This is likely the most challenging component of any strategy – its implementation. How much power the role is provided will speak volumes on how committed the current administration is to make sure these tools are ethical.
Minerva Tantoco is NYU McSilver Institute's chief AI officer and previously served as New York City’s first chief technology officer.
NEXT STORY: Editor’s Note: New York City is a migrant’s best hope for staying in the U.S.